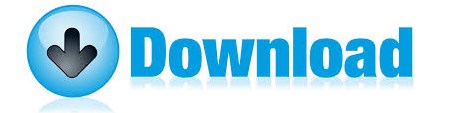
COCOMO MODEL ACADEMIC SOFTWARE
J Med Syst 39(11):1–20īarry WB, Ricardo V (2008) Achievements, and challenges in COCOMO-based software resource estimation. IEEE J Ambient Intell Humaniz Comput 10(2):661–668Īmin R, Islam SH, Biswas GP, Khan MK, Obaidat MS (2015) Design and analysis of an enhanced patient-server mutual authentication protocol for telecare medical information system. From the experiment, it is concluded that the proposed fuzzy-COCOMO model outperforms than the existing approaches in terms of relative error.Īlavalapati GG, Reddy R, Ashok KD, Vanga O, Awais A, Ji SS (2018) A privacy preserving three-factor authenticated key agreement protocol for client–server environment. Small or standard training sets were considered to deploy the estimate, and compare the performance with different estimators. A fuzzy-based analogy is obtained in the present study to select the nearest path from the history available to meet the project cost and time. Hence By combining more than one model estimates COCOMO and Gaussian Membership Function software estimate relative error will be the best suite. But, the COCOMO (COnstructive COst MOdel) gives an approximate estimate in terms of the month constant will not be same for simulating the study. Also, there is no existing approaches can do earlier prediction of the faults/errors or reduced misclassification rates. In earlier research works, various quality assurance metrics are used for analysing the SQ. Surprisingly there is no model-based architecture driven tool is available to intact the baseline estimates of the project based on the previous knowledge resource. More likely the metrics will not follow the standard protocol in terms of size, performance, technology and the complexity involved. By using these models, it is easy to identify the hurdles called as errors or faults Apriori to the development cycle. Software quality model (SQM) is highly concerned with standard metrics to qualify the software modules to classify bug or no bug. Therefore, this will positively encourage project managers to reuse the available LL knowledge – which will avoid old pitfalls and unleash hidden business opportunities.Software quality analysis and estimation is essential in developing a software to avoid faults and increase the reliability. It will eliminate the effort needed to manually search the LL repository. Conclusion: The automatic LL recall solution is valid with high accuracy. This is supported by a discerning accuracy of about 70% achieved in the case of top-k. Also, the results prove the success of using existing project artifacts to dynamically build the search query string. Results: Our case study results confirm the effectiveness of the automatic LL recall solution. Top-k and MAP well-known accuracy metrics are used as well. Furthermore, a real-world dataset of 212 LL records from 30 different software projects is used for validation.
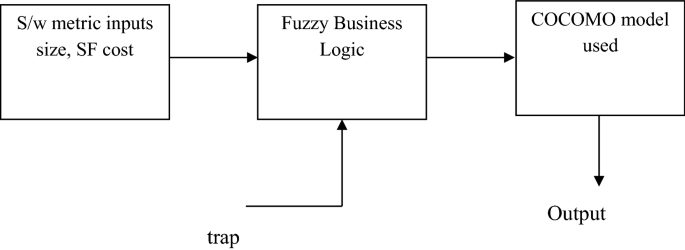
The study employs three of the most popular information retrieval models to construct the solution. An empirical case study is conducted to build the automatic LL recall solution and evaluate its effectiveness.
COCOMO MODEL ACADEMIC MANUAL
Method: We exploit existing project artifacts to build the LL search queries on-the-fly in order to bypass the tedious manual searching. This will dramatically save the time and effort of manually searching the unstructured LL repositories and thus encourage the LL exploitation. Objective: We aim to present a novel solution that provides an automatic process to recall relevant LL and to push those LL to project managers. This, in turn, can negatively affect the organization’s profitability and competitiveness. This can lead to the repetition of previous mistakes or even missing potential opportunities. However, manually searching this repository is a daunting task, so it is often disregarded.

LL are recorded within the organization repository for future reference to optimize planning, gain experience, and elevate market competitiveness.
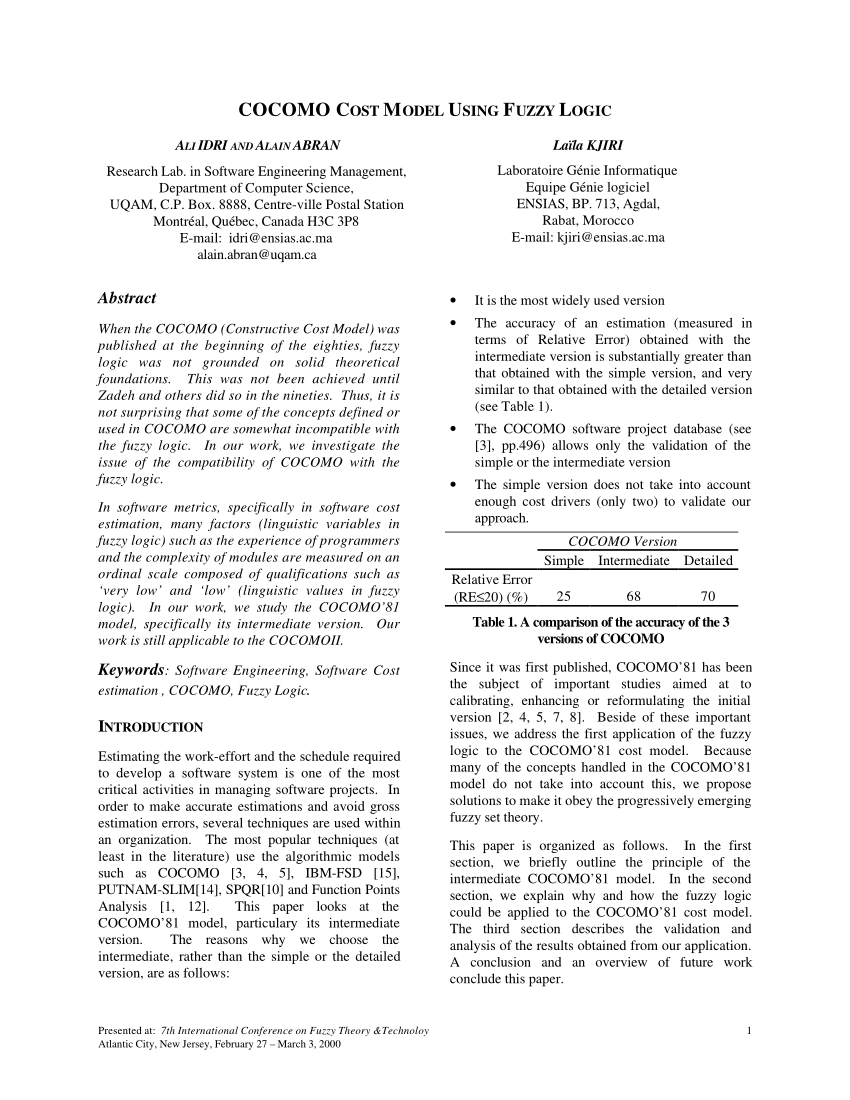
more Context: Lessons learned (LL) records constitute the software organization memory of successes and failures. Context: Lessons learned (LL) records constitute the software organization memory of successes an.
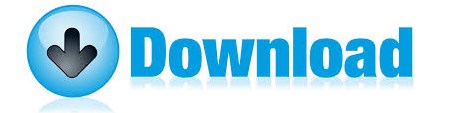